Event Description |
ASU Machine Learning Day
April 9, 2021
~ Technical Program ~
8:50 AM - 9:05 AM – Welcome and Opening Remarks (Dean Sandrin)
9:05 AM - 10:00 AM – Keynote Address Some Unique Problems with Social Media Data for Machine Learning and AI, Huan Liu, Arizona State University
10:05 AM - 11:05 AM – Ethics and ML
- Designing surveillance: The critical role of ethical frameworks in AI Games research, Florence Chee, Loyola University
- Excavating the "unintended consequences" of algorithms: The case of Facebook's "interest" classification system for ad targeting, Kelley Cotter, Arizona State University
- Anna Jobin, Alexander von Humboldt Institute for Internet and Society (Title TBD)
11:15AM - 12:15 PM – ML Thoery and Methods I
- Efficiently Evaluate Social Network Interventions, Johan Ugander, Stanford University
- A super scalable algorithm for short segment detection, Selena Niu, University of Arizona
- Designing disease-tracking metrics with causal machine learning, P. Richard Hahn, Arizona State University
12:15PM - 1:30PM – Virtual Poster Session
1:30PM - 2:30PM – ML and Social Science/Social Networks
- Resilience and adaptation in social networks, Will Hobbs, Cornell University
- Measuring physical and mental health using social media, Johannes Eichstaedt, Stanford University
- Erika Salomon, University of Chicago’s Center for Data Science and Public Policy (Title TBD)
2:45PM - 3:45PM – ML Thoery and Methods II
- Efficient active learning of halfspaces: noise tolerance and exploiting sparsity, Chicheng Zhang, University of Arizona
- Why does functional pruning yield such fast algorithms for optimal changepoint detection? Toby D Hocking, Northern Arizona University
- Optimizing for whom? The role of robustness in equitable algorithms, Kenneth Nieser, University of Wisconsin-Madison
3:45PM - 4:00PM – Research Computing
4:00PM – 4:05PM – Closing Remarks
You are invited to Arizona State University’s virtual Machine Learning Day.
Some Unique Problems with Social Media Data for Machine Learning and AI
Social media data is distinctive
from its traditional counterpart and opens the door for interdisciplinary
research and allows researchers to collectively study large-scale human
behavior otherwise impossible. The study of social media data brings about new
challenges for machine learning and data mining. In this talk, we will
introduce some unique issues of big social media data, e.g., the big data
paradox, the privacy-utility ‘trade-off’, and the evaluation dilemma. We will
also mention some efforts of using AI for good if time allows. With data
abundance and algorithmic development, we are better equipped than ever to
answer challenging and novel research questions and advance AI and CS.
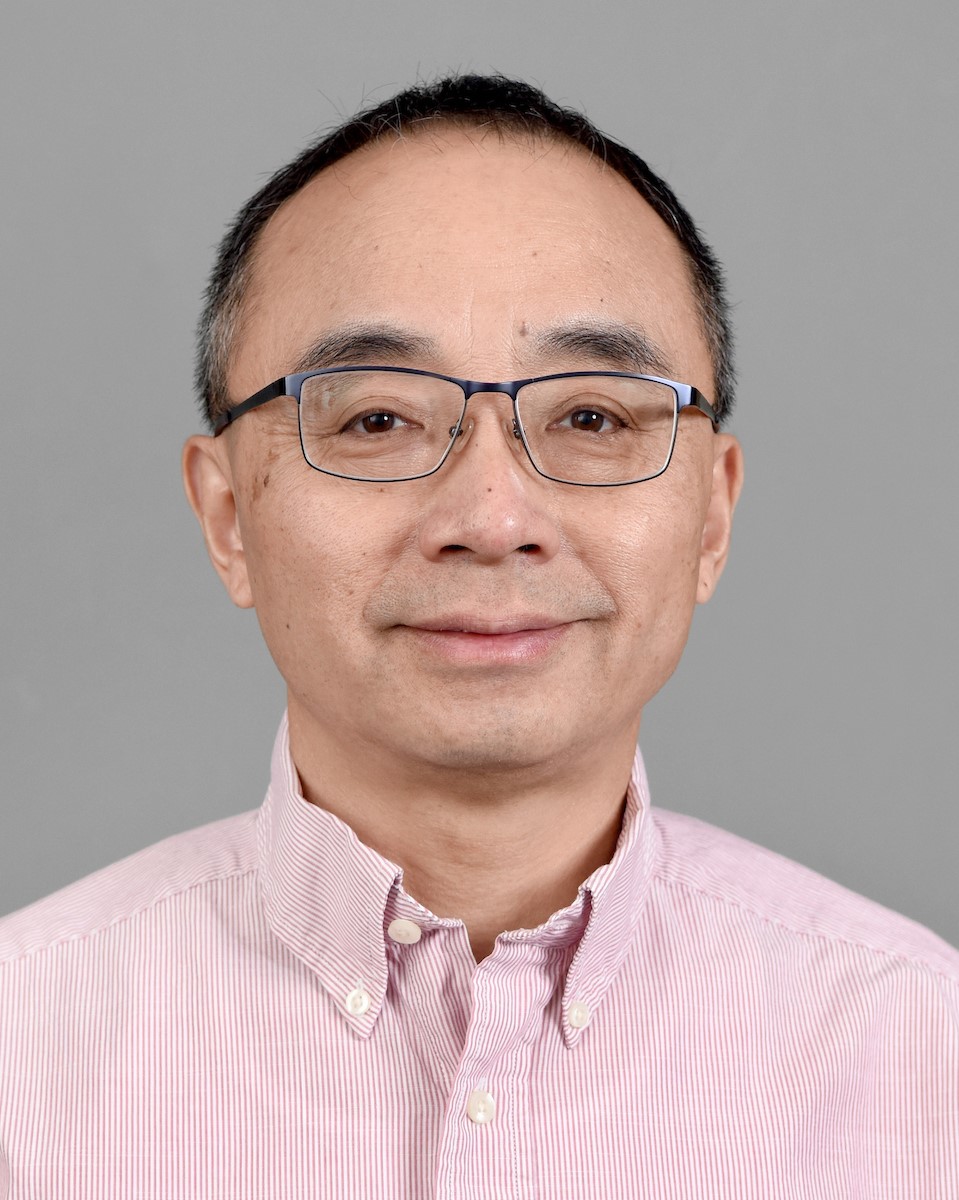
The events at Machine Learning Day include keynote speeches from top researchers in academic and industry, oral research presentations, and a virtual poster session. The topics of keynote addresses, presentations and posters cover the theory and practices of machine learning including:
- Adversarial machine learning
- Models, algorithms, and methods of machine learning
- Machine learning for recommendation systems
- Mathematical analysis and machine learning
- Practical applications of machine learning for cybersecurity and online privacy
- Statistical analysis and machine learning
- Event is FREE
- The event will be held on Zoom
- Registration will close once all spots are full.
- Registration is required
- Registration Open – January 2021
- Registration Closes – Once all spots are full or April 7, 2021
- Machine Learning Day – April 9, 2021